Using AIOps for Adaptive Incident Management
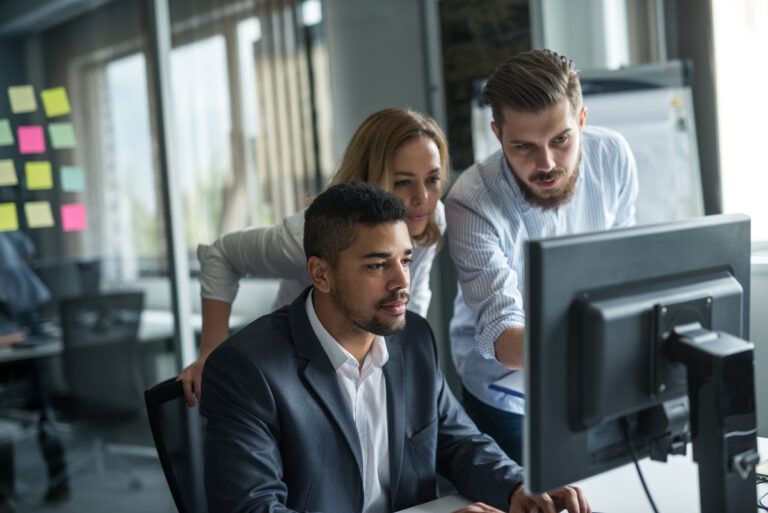
In the dynamic realm of IT, managing incidents swiftly and effectively is not just desirable—it’s essential. With the mounting complexity of IT infrastructures and the relentless evolution of cyber threats, traditional incident management approaches are struggling to keep pace. Enter AIOps, a groundbreaking approach that harnesses the power of artificial intelligence, machine learning, and automation to transform incident management into a more proactive and adaptive process.
Defining AIOps
AIOps, or Artificial Intelligence for IT Operations, revolutionizes the way IT operations are managed. At its core, AIOps integrates cutting-edge technologies like natural language processing (NLP), machine learning, and AI to automate and optimize various IT functions. This advanced technology provides deep insights into workflows, encouraging IT automation and ultimately streamlining incident management processes.
With AIOps, IT professionals can enhance their operations by leveraging sophisticated analytics to identify patterns, anomalies, and opportunities for optimization. This not only improves efficiency but also empowers teams to proactively address potential issues before they escalate into full-blown incidents.
Why Adaptive Incident Management Matters
In today’s fast-paced digital landscape, IT environments have become more complex and dynamic than ever. The widespread adoption of DevOps practices, microservices architectures, and continuous integration/continuous deployment (CI/CD) pipelines has introduced new challenges in monitoring and managing incidents. Incidents can disrupt services, tarnish user experiences, and damage reputations. This makes having an adaptive and flexible incident management strategy crucial for businesses striving to thrive in competitive markets.
Adaptive incident management allows organizations to respond swiftly to changing scenarios. By understanding the intricacies of their IT environments and deploying agile strategies, businesses can minimize downtime and ensure uninterrupted service delivery. Incorporating AIOps into this framework takes it a step further, enabling real-time insights and automated responses to emerging incidents.
Enhancing Incident Management with AIOps
Proactive Anomaly Detection
One of the standout features of AIOps is its ability to proactively detect anomalies that may signal potential incidents. By continuously monitoring performance metrics such as CPU usage, memory consumption, disk space, and network bandwidth, AIOps tools establish a baseline for normal operations. When these metrics deviate from the norm, AIOps algorithms such as Isolation Forest and Local Outlier Factor quickly flag anomalies, enabling IT teams to address issues before they escalate into major incidents.
Proactive anomaly detection empowers IT professionals to stay ahead of the curve. By identifying irregularities early on, teams can take preventive measures, thereby reducing the likelihood of service disruptions and ensuring a seamless user experience.
Leveraging Historical Data for Faster Resolution
The historical record of past incidents is a valuable resource for improving incident response strategies. AIOps tools can mine this data to identify patterns and similarities between past and current incidents. By employing machine learning techniques like K-means clustering, AIOps suggests the most effective response based on historical data. This capability accelerates incident resolution and equips teams with the tools they need to tackle recurring issues efficiently.
Analyzing historical data fosters a culture of continuous improvement within IT teams. By learning from past incidents, organizations can refine their incident management processes, enhance their decision-making capabilities, and mitigate the impact of future incidents.
Automated Team Notifications
Timely notification of relevant teams is critical for effective incident management. AIOps tools automate this process by collating essential incident information and sending intelligent alerts to the appropriate teams. This automation ensures no critical incidents are overlooked and helps reduce alert fatigue by filtering out non-actionable alerts.
Automated notifications streamline communication and collaboration among IT teams, enabling them to respond promptly to incidents and implement solutions swiftly. By eliminating manual processes, organizations can focus on what matters most—resolving incidents and maintaining optimal system performance.
Streamlining Incident Reports and Communication
During major incidents, organizations can be overwhelmed with support tickets and communication needs. AIOps tools leverage NLP algorithms to sort and categorize tickets, quickly identifying root causes and enabling faster resolution. This streamlined process not only improves response times but also enhances communication between teams.
Efficient incident reporting and communication are cornerstones of successful incident management. By automating these processes, AIOps empowers IT teams to allocate their resources more effectively, improving overall productivity and strengthening cross-functional collaboration.
Root-Cause Analysis and Optimization
Determining the root cause of an incident can be a time-consuming process. AIOps tools expedite this by correlating logs and metrics using unsupervised machine learning techniques. By consolidating data from various sources into a centralized dashboard, AIOps provides actionable insights that help teams identify and address root causes efficiently. This proactive approach also helps prevent future incidents by identifying optimization opportunities.
Root-cause analysis lays the foundation for continuous improvement within IT operations. By pinpointing the underlying factors contributing to incidents, organizations can implement targeted solutions that enhance system stability and prevent recurrence.
The Importance of Real-Time Responses and Proactive Monitoring
Today’s digital environment requires real-time responses and proactive monitoring. AIOps enables IT teams to remain agile and responsive, automating repetitive tasks and ensuring accurate, up-to-date information is always available. By intelligently sorting and correlating data, AIOps helps teams focus on high-risk incidents, improving overall incident management and reducing downtime.
Real-time responses empower organizations to maintain a high level of operational resilience. By preemptively addressing potential issues, IT teams can mitigate risks, safeguard critical systems, and provide consistent service delivery to their customers.
Take Your Incident Management to the Next Level
Incidents are an inevitable part of any digital operation. However, with the right tools and strategies, organizations can manage them effectively and minimize their impact. AIOps for incident management offers a powerful solution, providing the flexibility and adaptability needed to handle today’s complex IT environments. By proactively identifying issues, automating notifications, and leveraging historical data, AIOps enables teams to respond quickly, efficiently, and confidently to any incident.
For IT professionals, DevOps teams, and operations managers seeking to enhance their incident management strategies, AIOps presents a compelling opportunity. By integrating AI-enabled incident management practices into your organization, you can unlock a new level of efficiency, resilience, and competitive advantage.
Ready to transform your incident management strategy? Download the new AIOps Buyer’s Guide and discover firsthand how AIOps can revolutionize your approach to incident management. Explore the future of IT operations and empower your teams to thrive in an ever-evolving digital landscape.