How to Use Big Data to Your Advantage
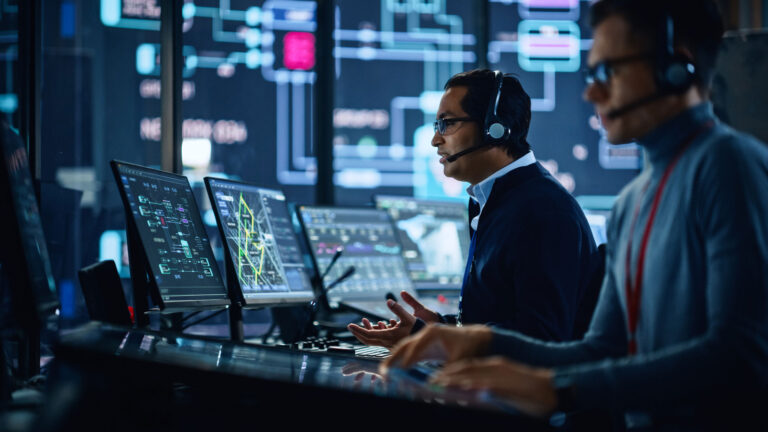
Users have been generating increasing amounts of data in the past few years, partly due to rapid digitalization since the pandemic. As a result, increasing numbers of analytics applications are capitalizing on these data assets.
However, building scalable systems is no trivial task and incidents are inevitable. Complex systems generate data in the form of logs, traces, metrics, and more, which organizations often find themselves sprinting through. Such logs are a powerhouse of valuable information. When analyzed with third-party datasets, these can help the IT operations team identify the incident lineage.
Extracting Incident Management Insights From Big Data
Sophisticated AI algorithms and powerful infrastructure level the playing field. Consequently, speed differentiates businesses with a competitive edge. The pace of innovation frequently calls for users to migrate centralized IT assets to modern microservice-based systems. However, these complex systems are as unpredictable as centralized systems. Furthermore, they require advanced tools in a changing environment.
Analyzing vast amounts of data is a herculean task. Data assets can quickly become a liability if they’re not well managed. This is where incident management systems come in. An effective incident management system improves the incident lifecycle and helps the IT operations team understand why outages occur.
The Challenges of Working With Big Data
The rapid increase in data from disparate sources makes it impractical for people to generate their own insights. According to Miller’s law, the average person can only hold about seven objects in their working memory. This means that the human brain cannot analyze vast datasets to generate actionable insights. However, artificial intelligence (AI) and machine learning (ML) algorithms can overcome this limitation. These algorithms can uncover hidden data patterns, empowering businesses with data-driven insights.
An incident signifies an outage or a negative impact on business services. Incident logs store all the relevant details, including the impacted application, its connected services, timestamp, severity, and so on. These logs generate large amounts of vital data from different IT infrastructures, services, applications, and networks to detect faults early. However, the low signal-to-noise ratio of such a data set critically limits its analysis.
First, if the data set has a low signal-to-noise ratio, it’s difficult for IT engineers to identify the subset of data relevant to troubleshoot the error logs. Secondly, such incident logs are largely imbalanced since most data capture the system’s healthy state. Only a small subset of data carries phenomena related to incidents that must be filtered and monitored for effective incident response management.
When incidents occur, engineers must expend precious brainpower to examine them manually. This makes a clear case for using AI and ML algorithms to manage large-scale datasets and generate actionable insights.
What Is Artificial Intelligence for IT Operations (AIOps)?
Observability will be the data industry’s next big focus, allowing you to see the health of your data pipelines. It’s a big step up from the black-box testing approach, which only provides limited information about output deviations concerning changes at the input.
AIOps that use AI and ML algorithms empower IT operations. AIOps equips the IT team with the necessary support to efficiently and effectively manage systems at scale. For example, AIOps automatically analyzes data to maintain system health. This removes the risk of manual errors or delinquencies.
AIOps systems save significant time for IT teams by automatically resolving alerts. AIOps can aggregate and correlate patterns from data sources like storage, infrastructure, and network. ML algorithms generate data-driven inferences from large data sets by:
- Predicting when the next outage will be
- Identifying inefficiencies
- Predicting which type of outages are most likely to occur
- Analyzing the root cause of incidents
These insights give the operations team the details necessary to respond to incidents, leading to quicker incident resolution. AIOps breaks down the information silos restricting information to a limited group of engineers. This democratization of knowledge empowers more team members to address incidents.
AIOps is critical to faster incident management and reducing downtime, keeping customer and system expectations in check.
AIOps and ML Capabilities
Utilizing ML algorithms that use IT data and telemetry data, AIOps builds a predictive maintenance arm for the systems. Advanced techniques such as natural language processing (NLP) analyze unstructured data, like error logs, outage sources, defect types, incident categories, and event descriptions. As computers don’t understand raw text data, NLP converts the natural language data into a vectorized form. Computers then apply the processed data in many cases, such as clustering similar incidents using unsupervised algorithms. Such capabilities can detect issues in your services early, reducing the impact on consumers downstream.
As operations grow, incident logs can quickly proliferate and swarm the operations team with alerts. It’s challenging to filter out false alerts—also known as false positives—and irrelevant data wastes time. AIOps solutions cut through the noise. Sophisticated algorithms discover telemetry data patterns to identify the cause of outages and alert the site reliability engineering (SRE) engineer. Consequently, engineers can focus on what’s important, like text data, timestamps, and the source of incidents.
AIOps allows an autonomous workflow that resolves some bugs without human intervention. Engineers can either fix the other bugs themselves or follow a prescribed resolution.
Insights From Big Data
Static rules are insufficient for monitoring modern IT infrastructure’s complex, modular, and distributed nature. The operations team can only detect issues they’ve previously encountered. And since issues don’t always follow a repeated pattern, it’s difficult for the operations team to detect and resolve new issues swiftly with minimal business disruption.
AIOps can prevent outages by alerting the team to issues they’ve never encountered before. However, AIOps isn’t just about alerting the team to existing issues. It also promotes learning and recording fixes for every incident. Information on the nature of incidents, proposed resolutions, and final working solutions become training data for ML algorithms. A tightly-coupled feedback loop allows the algorithms to learn and identify underlying causes to help prevent similar incidents in the future.
Conclusion
To build trustworthy systems, you must be able to verify whether your system is behaving as intended. This requires you to analyze large data sets with the help of an AIOps system that leverages sophisticated algorithms. To increase efficiencies at every layer of the incident management system and tackle the causes of recurring incidents, your organization needs an AIOps component.